Faster than simulated annealers and rivaling the performance of quantum annealers, the Fujitsu Co-Creation Research Laboratory applies quantum-inspired Digital Annealer technology to solving combinatorial optimization problems.
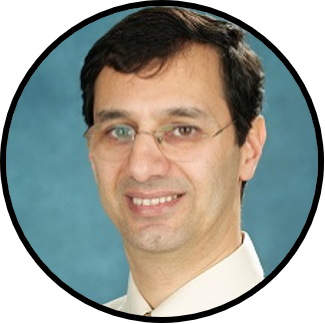
Professor Ali Sheikholeslami
My group works on the intersection of algorithms, architecture, and hardware implementation of CMOS annealers and samplers, and strives to improve the size (number of binary variables that can be accommodated), speed (reducing time to solution), energy efficiency (energy per operation) and flexibility (the ability to trade off between speed and size), all given the hardware resource constraints.
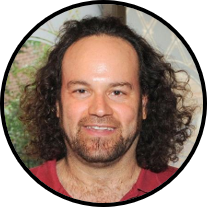
Professor Jeffrey Rosenthal
We study the theoretical and mathematical properties of Markov chain Monte Carlo (MCMC) and annealing algorithms. We are investigating the ways in which the DA uses these algorithms, and how they can be improved to produce more efficient optimizations and more accurate samples. We have created a Javascript web page to illustrate some of these issues, which can be viewed at: http://probability.ca/metropolis
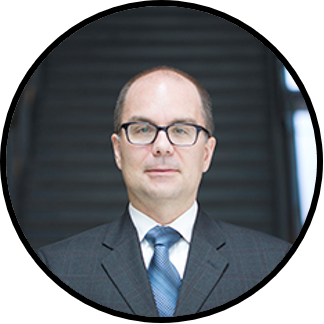
Professor Christopher Beck
Our research on the DA focuses on understanding how it can be used to solve hard combinatorial problems in Artificial Intelligence and Operations Research. We are interested in a variety of problem classes (e.g., constraint programs, SAT) and applications such as scheduling and wind farm layout optimization. We will seek to use the DA to find exact solutions through hybridization with existing exact optimization methods and to expand the size of problems that can be addressed through systematic decomposition frameworks.
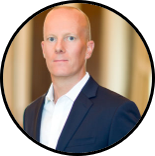
Professor Edward Sargent
Our research focuses on the application of DA for material design specifically to optimize components and ratios of high-entropy alloys for water splitting using DA. We are developing a machine learning method with uncertainty prediction in the form of quadratic unconstrained binary optimization (QUBO). Based on this machine learning method, we are designing high-entropy alloys for water-splitting catalytic materials using active learning and realizing the designed catalysts experimentally.

Professor Jason Anderson
To be completed
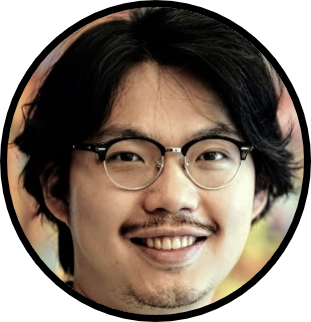
Professor Jimmy Ba
To be completed